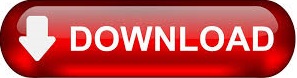
How concerned should you be if your model isn’t perfect, if your residuals look a bit unhealthy? It’s up to you. How much does it matter if my model isn’t perfect? If the points skew drastically from the line, you could consider adjusting your model by adding or removing other variables in the regression model. This chart displays the standardized residuals on the y-axis and the theoretical quantiles on the x-axis.ĭata that aligns closely to the dotted line indicates a normal distribution.
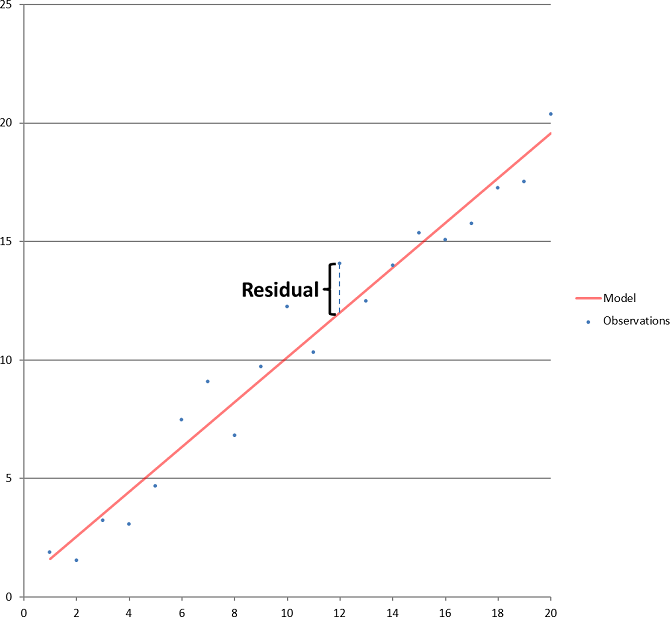
Normal Q-Q Residual Plot:Ĭlick Show Normal Q-Q residual plot to display a Q-Q plot assessing data skew and model fit. In a second we’ll break down why and what to do about it. If you can detect a clear pattern or trend in your residuals, then your model has room for improvement. These plots aren’t evenly distributed vertically, or they have an outlier, or they have a clear shape to them. Here’s some residual plots that don’t meet those requirements: (3) in general, there aren’t any clear patterns. (2) they’re clustered around the lower single digits of the y-axis (e.g., 0.5 or 1.5, not 30 or 150). (1) they’re pretty symmetrically distributed, tending to cluster towards the middle of the plot. Ideally your plot of the residuals looks like one of these: …positive values for the residual (on the y-axis) mean the prediction was too low, and negative values mean the prediction was too high 0 means the guess was exactly correct. The distance from the line at 0 is how bad the prediction was for that value. In the plot on the right, each point is one day, where the prediction made by the model is on the x-axis and the accuracy of the prediction is on the y-axis. (Stats iQ presents residuals as standardized residuals, which means every residual plot you look at with any model is on the same standardized y-axis.) The most useful way to plot the residuals, though, is with your predicted values on the x-axis and your residuals on the y-axis. More often, though, you’ll have multiple explanatory variables, and these charts will look quite different from a plot of any one explanatory variable vs. ” That’s common when your regression equation only has one explanatory variable. “Revenue” charts above them, but the x-axis is predicted “Revenue” instead of “Temperature. Note that these charts look just like the “Temperature” vs. The model for the chart on the far right is the opposite the model’s predictions aren’t very good at all. So instead, let’s plot the predicted values versus the observed values for these same datasets.Īgain, the model for the chart on the left is very accurate there’s a strong correlation between the model’s predictions and its actual results. It’s clear that for both lemonade stands, a higher “Temperature” is associated with higher “Revenue.” But at a given “Temperature,” you could forecast the “Revenue” of the left lemonade stand much more accurately than the right lemonade stand, which means the model is much more accurate.īut most models have more than one explanatory variable, and it’s not practical to represent more variables in a chart like that. In a simple model like this, with only two variables, you can get a sense of how accurate the model is just by relating “Temperature” to “Revenue.” Here’s the same regression run on two different lemonade stands, one where the model is very accurate, one where the model is not:
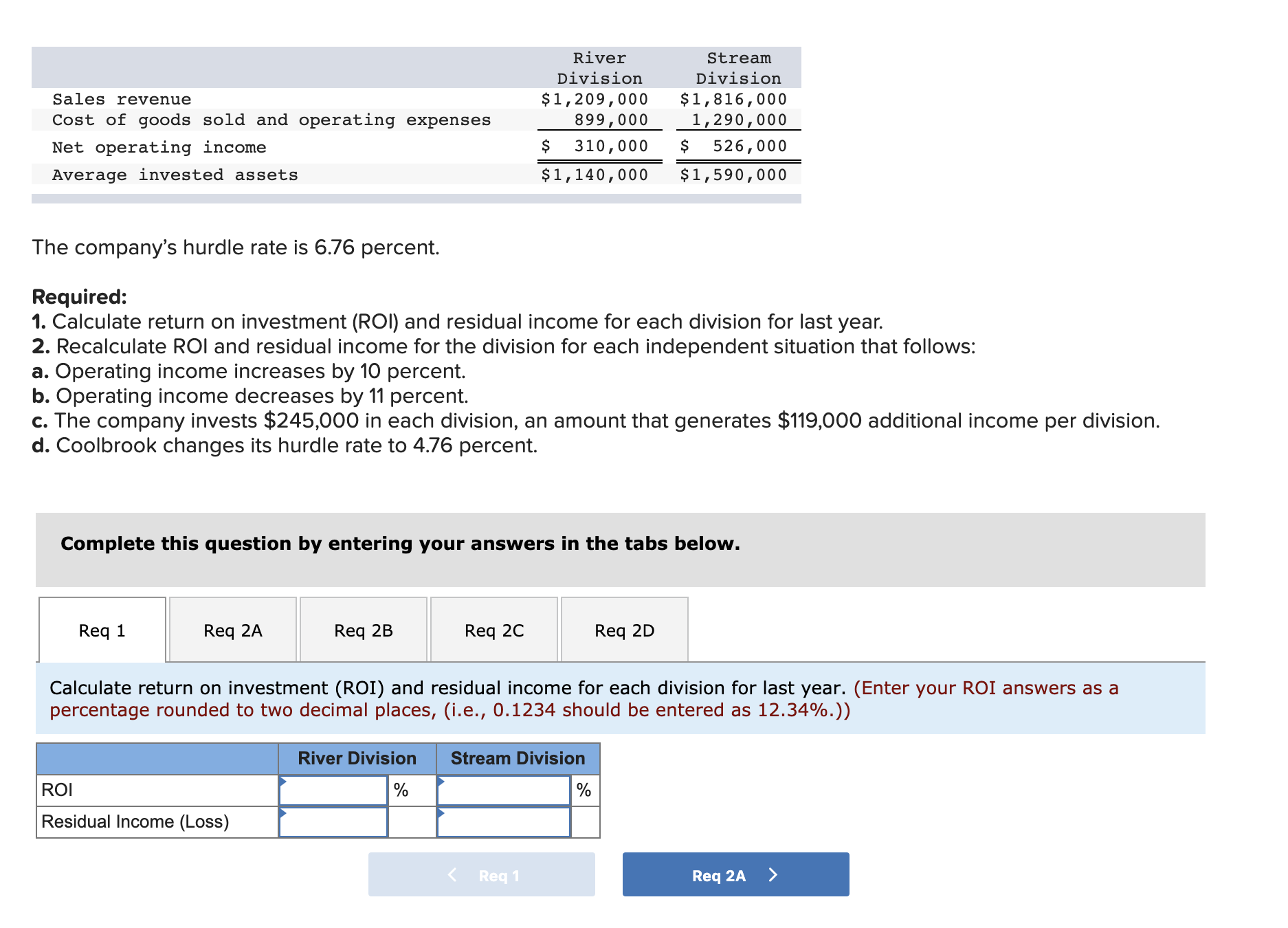
We’re going to use the observed, predicted, and residual values to assess and improve the model. You can imagine that every row of data now has, in addition, a predicted value and a residual. The residual is the bit that’s left when you subtract the predicted value from the observed value. In this case, the prediction is off by 2 that difference, the 2, is called the residual. Your model isn’t always perfectly right, of course. That’s the predicted value for that day, also known as the value for “Revenue” the regression equation would have predicted based on the “Temperature.” So if we insert 30.7 at our value for “Temperature”… That 50 is your observed or actual output, the value that actually happened. Let’s say one day at the lemonade stand it was 30.7 degrees and “Revenue” was $50.
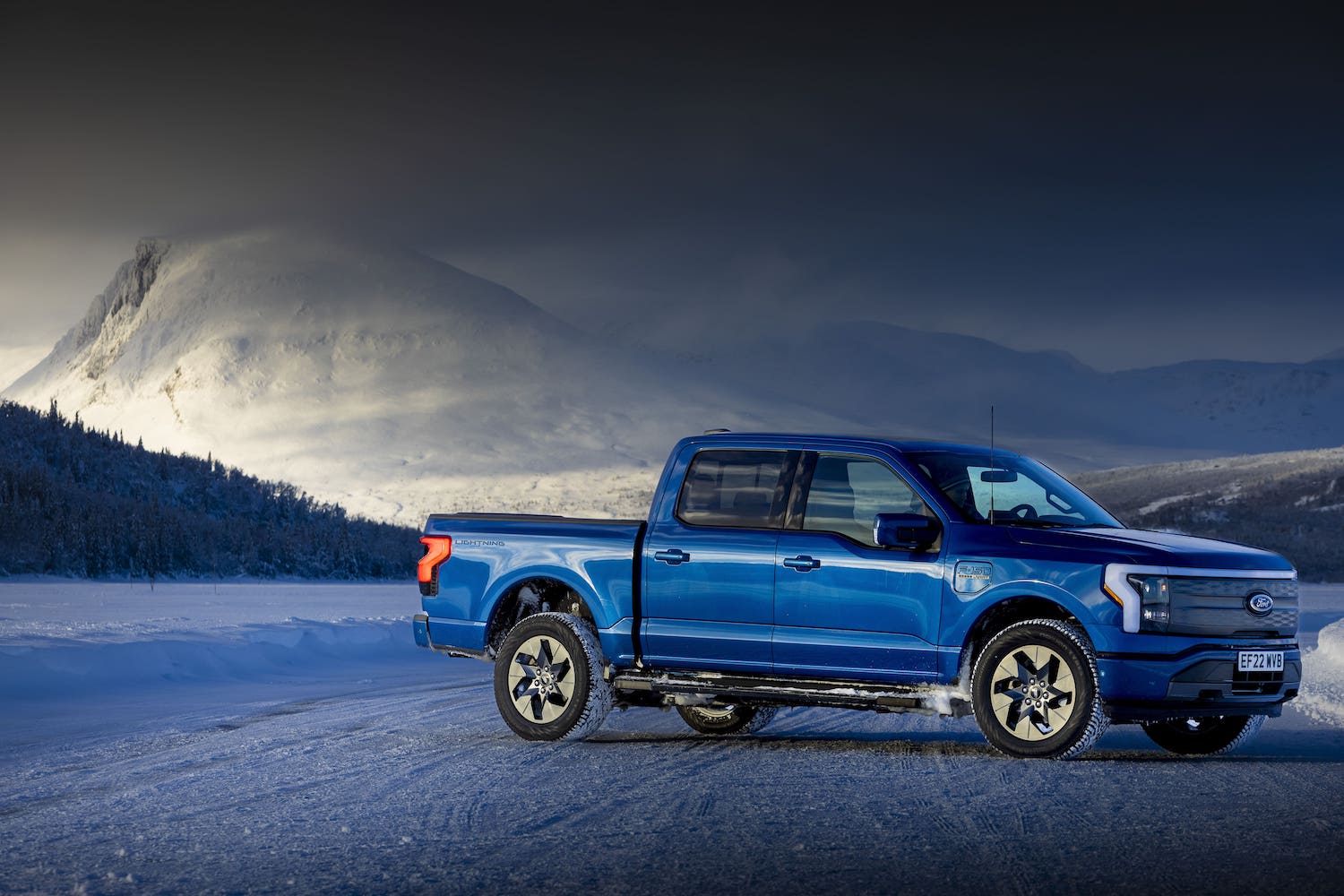
Since this residual is very close to 0, this means that the regression line was an accurate predictor of the daughter's height.The regression equation describing the relationship between “Temperature” and “Revenue” is: Therefore the residual for the 59 inch tall mother is 0.04.
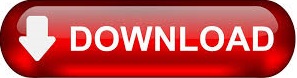